Project Summary: Loan Portfolio Analysis and Machine Learning
Overview: This project involved a detailed analysis of a large loan portfolio dataset containing over 9,000 entries, each representing individual loans with various attributes such as loan status, interest rates, country of issuance, and repayment details. The primary goals were to explore patterns in loan repayment, analyze the impact of interest rates on repayment, and identify trends in loan issuance across different countries and time periods. To achieve these goals, I employed various data analysis techniques and machine learning models.
Data Analysis:
Loan Status Distribution: An initial exploration of the data revealed the distribution of loan statuses, with a significant number of loans being fully repaid. Visualizations were created to better understand the distribution of current loan statuses as of June 30, 2024.
Interest Rate Analysis: The interest rates of the loans were analyzed for patterns and outliers. The data revealed a wide range of interest rates, with most loans falling below 1%. Skewness was addressed by applying a log transformation to the interest rates.
Repayment Duration: The average repayment duration was calculated and analyzed by country, revealing significant variations in repayment timelines across different regions.
Machine Learning Models: To gain deeper insights and predict loan outcomes, I employed several machine learning models:
Logistic Regression:
Purpose: To predict whether a loan would be fully repaid based on its interest rate.
Performance: The logistic regression model achieved an accuracy of 86%, indicating a relatively strong predictive power for classifying loans as fully repaid or not.
OLS Regression:
Purpose: To explore the relationship between interest rates and loan repayment. The model showed a positive relationship, with higher interest rates correlating with an increased likelihood of repayment, although the R-squared value indicated that interest rates alone do not fully explain repayment behavior.
Decision Tree Classifier:
Purpose: To classify loans based on various attributes and predict their repayment status.
Performance: The decision tree model provided a detailed breakdown of classifications, although the accuracy was relatively low, suggesting that more complex models or additional features might be needed for better predictions.
Key Findings:
Interest Rate and Repayment: There is a positive relationship between interest rates and loan repayment, but interest rates alone are not a strong predictor of whether a loan will be fully repaid.
Country-Specific Trends: Certain countries showed a higher propensity for quick repayment, while others had more prolonged repayment durations. Countries like Indonesia, Brazil, and Mexico were among those with the highest number of fully repaid loans.
Loan Issuance Trends: The data indicated specific periods with higher loan issuance, and a weak correlation was observed between the number of loans signed and the average interest rates during those periods.
Loan Sales to Third Parties: Approximately 9.58% of loans were sold to third parties, highlighting a significant portion of the portfolio that was transferred away from the original lenders.
Conclusion: This project provided a comprehensive analysis of the loan portfolio, offering insights into repayment behaviors, interest rate impacts, and country-specific loan trends. The findings from the machine learning models and data analysis will help inform strategies for managing future loan portfolios and understanding factors that influence loan repayment.
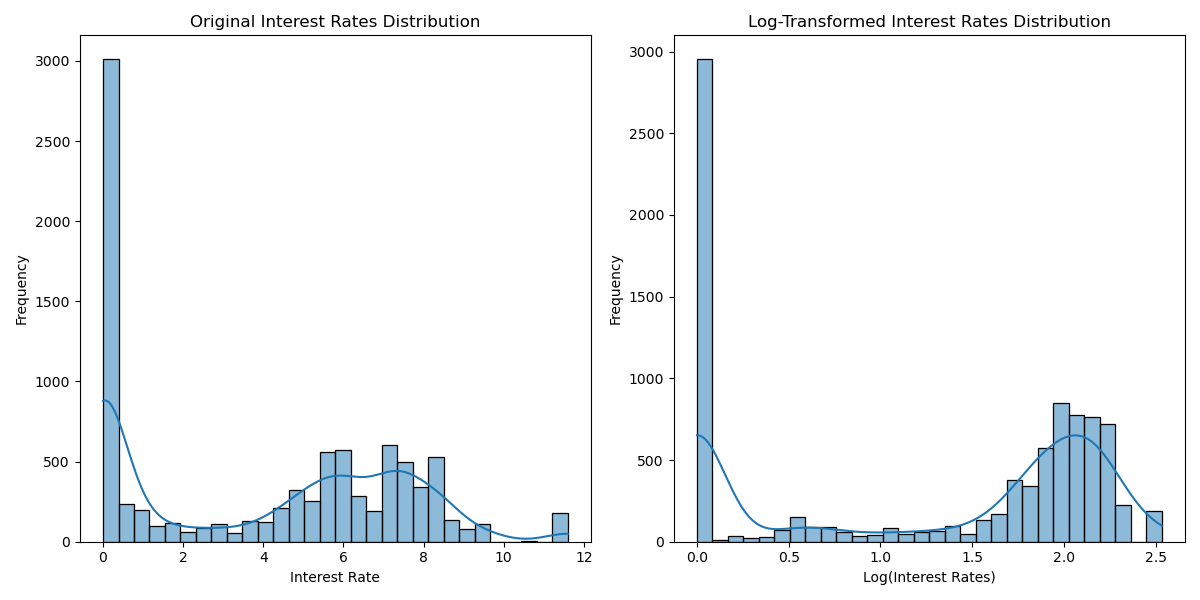
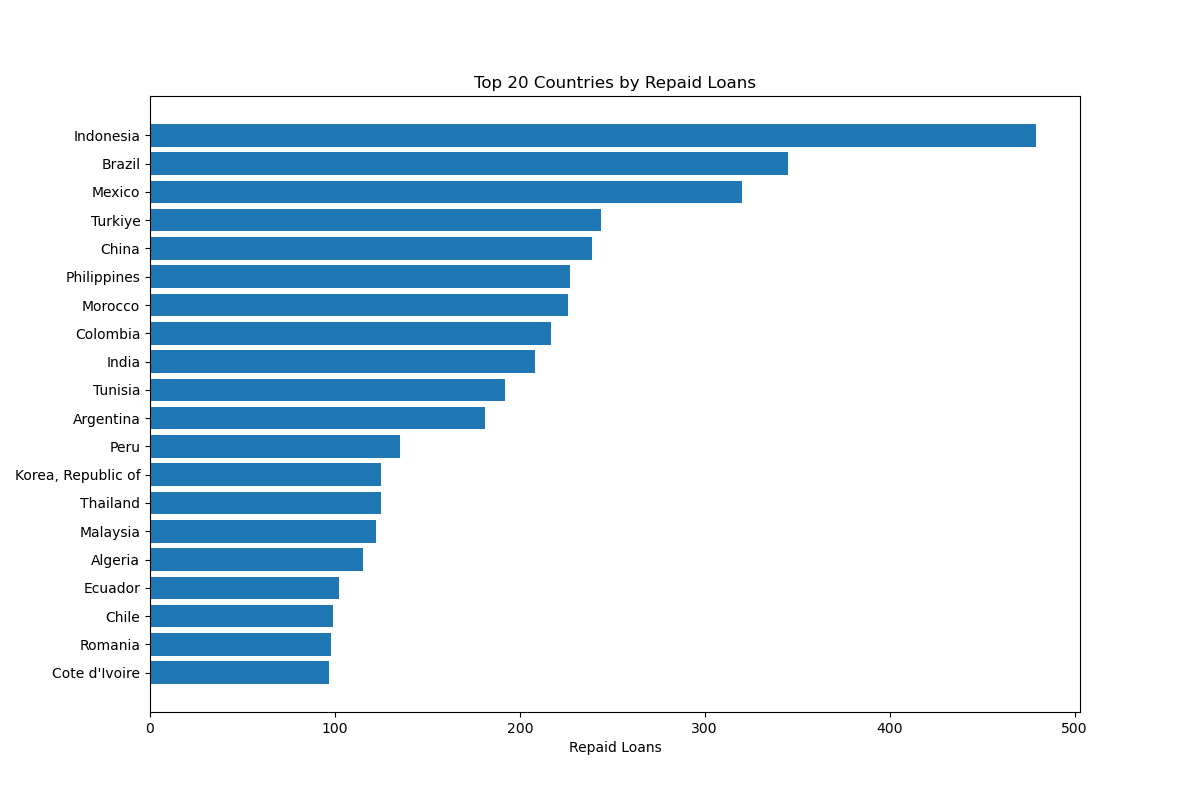
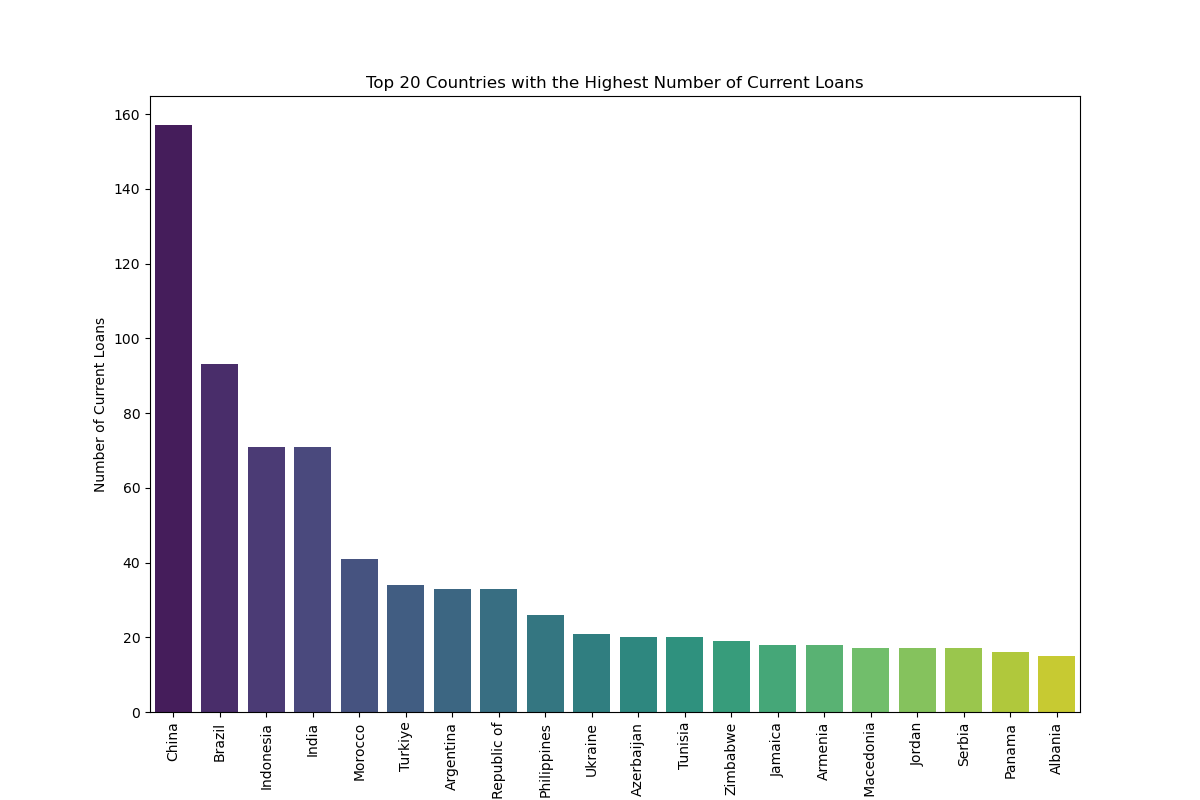
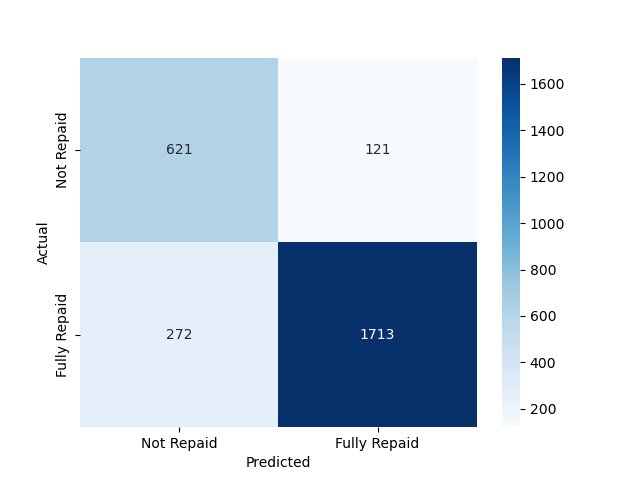